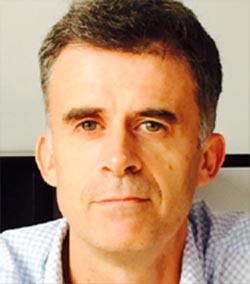
Frédéric Saunier
Managing Director, Diapason
Techniques related to Artificial Intelligence find several applications in the field of treasury. They improve processes related to cash flow forecasting, bank reconciliation, hedging of foreign exchange risks, and fraud prevention. In addition to the improvement of traditional functionalities linked to treasury and market risk management, the future of TRMS (Treasury & Risk Management Systems) necessarily involves the application of these techniques to the treasury business.
It is naturally not a question of substituting these technologies for the know-how of treasurers and market operators, but on the contrary of providing them with control and decision-making aid tools, which, moreover, will limit operational risk and facilitate carrying out certain time-consuming and low value-added tasks.
Getting Artificial Intelligence off the ground in the world of Corporate Treasury
The existing techniques are not recent. Nevertheless, their democratization in the treasury professions has found little concrete application to date. It is therefore absolutely necessary to carry out educational work, both on the technical level and on the need to access quality data, and on its impact on human resources. The objective of these processes is to provide tools for control and refinement, but in no way to replace humans. A parallel can be drawn with electronic trading, which has not been able to take into account certain market accidents. It is the combination of AI and critical human insight that will optimize processes.
Moreover, the need to have exhaustive and quality information can be considered as a hindrance to the launch of a project of this type. A preliminary study to list the relevant data sources is a prerequisite for any AI project
That said, the application of Artificial Intelligence processes makes the entire processing chain more reliable, and provides decision support tools that are difficult to access with traditional methodologies. AI is thus applied to modeling cash flow and foreign exchange position forecasts, detecting fraud and optimizing bank reconciliation.
Treasurers know that the quality of a liquidity and exchange position forecast is a prerequisite for setting up a financing and hedging policy. In other words, reliable data helps avoid the costs associated with bad decisions in terms of financing or coverage.
With our Diapason solution, we are currently in an experimental phase. Several customers are associated with this experiment, within the framework of a model that we produce jointly on the basis of their data.
Experience shows that the data cleaning phase is the most delicate and time-consuming step in the process.
Promising initial results have nevertheless made it possible to obtain low margins of error between the results obtained by the models and the cash flows recorded.
The application of AI to forecasting processes
It is necessary when talking about Artificial Intelligence to distinguish between the different levels of existing automation:
Rules engines that execute a set of business operations in a specific context. These are usually deterministic algorithms with a predefined rule base.
Machine Learning is based on learning techniques and makes it possible to adapt the parameters of the system to the data processed. In the context of cash flow forecasts, the data processed is structured and the classic ML algorithms (especially for the analysis of time series) provide satisfactory results.
In addition, Deep Learning techniques can be used upstream of the forecast to extract, for example, the explanatory factors, for the selection of models or in cases where the performance of conventional algorithms is insufficient.
However, it often remains necessary to add exogenous elements to the model in order to issue more relevant forecasts. The data needed to establish a cash flow forecast is in fact made up of endogenous data (at a minimum, past cash flows) and potentially exogenous data (for example, seasonality indicators, or any market data having an impact on operational activity).
Any information, endogenous or exogenous, can in theory be used to perform predictive analysis. Nevertheless, experience shows that collecting this information, which comes from a wide variety of sources, and often in an unstructured way, represents the most complex phase of an AI project. In addition, access to exogenous data can be complex, even costly.
Other areas of treasury covered by AI
Cash reconciliation is a feature that can greatly benefit from the self-learning techniques offered by Artificial Intelligence. Indeed, the history of reconciliations performed is a valuable database for suggesting automatic reconciliation behaviors.
The determination of the foreign exchange position (partly based on cash forecasts), as well as the optimization of the hedging ratio are also areas where the contributions of Artificial Intelligence are considerable. Increased reliability of the foreign exchange position makes it possible to optimize the hedging to be put in place, and to reduce the foreign exchange risk, while limiting the costs induced by any over-hedging.
Finally, fraud detection is currently mainly handled by rule engines (which identify atypical behavior). The contributions of Artificial Intelligence will go beyond this approach, in particular by limiting the false positives generated by existing methods (of the rule engine type), and therefore costly manual searches.
The main stages of an AI implementation project in cash flow forecasts
For a predictive analytics project in the field of cash flow forecasting (or for any project relying on time series analysis):
The first step, which is undoubtedly the most complex, consists of listing all the necessary data sources (both endogenous and exogenous).
This may be the TRMS (cash and risk management system), the ERP (integrated management software package), the information system of the “financial consolidation” function, statements of accounts, etc., for endogenous data. And from external sources for exogenous data.
Once this data has been identified and consolidated in a data warehouse, it is necessary to clean it (add missing data, remove redundant and aberrant data)…, then to homogenize it.
These data must be subject to visualization processes, allowing them to be apprehended in a concrete way (visualization according to the main explanatory variables composing them), before an advanced analysis phase (in terms of seasonality, correlations, etc.).
The modeling step can then start.
Just as there is no single transformation methodology, there is no single modeling technique. Some techniques may be more or less effective depending on the data. The proposed solution must be able to suggest the most efficient model based on the profile of the data analyzed.
Finally, the models based on self-learning techniques will evolve according to new data feeding the database.
Exceptional events, such as COVID-19, or a one-time external growth operation cannot be anticipated through a model. The intervention of the business expert is decisive at this level to correct these limits (when possible).
It is also essential to have “back testing” and “out of sample” analysis processes (analysis of errors based on a retraining process carried out on real data) so as not to introduce bias into the model.
In all cases, the experience of the treasurer and his critical eye on the results provided by the models are essential to detect possible flaws in the modelling.
Key success factors for an implementation project
- The first key to success depends on the quality of the data. According to the popular adage in the data science world, “garbage in, garbage out”: relying on incorrect data produces an incorrect result. It is therefore absolutely necessary to ensure that you have exhaustive data, cleaned of outliers or redundant values, reproducing the missing data. Thus, once the data sources have been identified, we support the client in determining the missing, outlying data, and redundant, and exogenous factors. This phase requires strong interaction with the client, who alone is capable of judging the quality of this data. The second stage consists of adapting and transforming the data, and creating the explanatory factors necessary for preparing the data that will be modeling.
- The second key success factor is based on the involvement of business teams. It is indeed illusory to think that the machine can replace human experience. The critical eye of the business expert is fundamental, both in the analysis of the data upstream of the project, and in the control or potentially the correction of the results obtained. We can never repeat enough that these techniques do not replace humans, but are intended to help them in the quality and speed of their decision-making.
In the end, the results of our work already show that Artificial Intelligence promises to be a valuable decision-making tool for treasurers. In addition to saving them time on a daily basis and helping to secure certain processes, this new tool will make it possible to avoid significant costs linked to over-financing, over-hedging, or exchange losses linked to poor anticipation of the position.
About the Author
Frédéric Saunier, Chief Executive Officer
With over 25 years of expertise in financial services, Frédéric Saunier advises Diapason’s clients on the digitalization of their financial departments. As an expert in Artificial Intelligence, Blockchain, Financial Flow Security, and Sustainable Development (ESG), Frédéric shares his knowledge through his articles.